Statement from An de Wispelaere
In this article, the Chief Product Officer (CPO) of our company, An de Wispelaere, addresses some key questions regarding the use of artificial intelligence (AI) in route optimization. As AI gains more popularity in software development, An provides valuable insights into its advantages, limitations, and risks. In addition, she explains how PTV Logistics integrates academic research with its own studies to create innovative route optimization solutions within the vehicle routing problems (VRP) field that surpasses industry standards. Keep reading to discover more about the potential benefits and drawbacks of using AI or reinforcement learning in VRP.
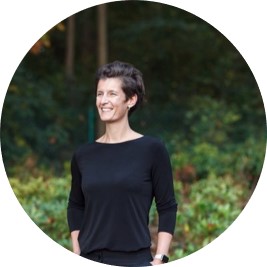
Q: Will PTV Logistics use AI or reinforcement learning?
Answer An: Our fundamental objective is to continuously innovate and maintain a competitive edge. In pursuit of this goal, we are committed to leveraging all available methods and cutting-edge techniques, including AI or reinforcement learning. It should always be superior to other techniques that we use. Following the merger of PTV and Conundra, we are poised to enhance our innovation efforts and further outpace the competition.
Take control of your logistics with our powerful SaaS route optimization software OptiFlow.
Q: What are the Pros of AI in VRP Context?
Answer An: AI can be used extensively in the Learn area. (Learn = using the execution data to gain insights on and improve the quality of our route optimization plan, by enriching the master data used to create the plan). For example, AI and Machine learning is used to analyze customer delivery times which might be time-dependent and even driver/resource/location related.
Geocoding: AI is a powerful tool for improving the accuracy and efficiency of geocoding, helping to enable more accurate and effective mapping and location-based services. Examples are:
- Address Parsing: AI can be used to parse the individual components of an address, such as the street name, city, state, and zip code. This parsing can help improve the accuracy of the geocoding process by ensuring that each component is correctly identified and matched to its corresponding geographic location.
- Natural Language Processing: AI can also be used to interpret natural language inputs, such as handwritten or spoken addresses. Natural language processing (NLP) can help identify and correct errors or ambiguities in the address, such as misspellings, missing or incorrect components, or unclear abbreviations.
Service Levels – Assessing service levels based on customer proximity to the depot is important. Delivery cost is obviously related to distance, but density in the delivery area also plays a crucial role. Accurately forecasting the entire delivery network of a particular day is necessary to make on-the-fly estimations or predictions of specific delivery costs for a customer and timeslot. AI techniques have a proven track record in forecasting and are highly relevant in this context.
Planning robustness: Road transport is prone to real-time changes, such as traffic congestion or sudden changes in demand. Machine Learning algorithms can learn from past data and predict future trends, which can help in adjusting the routes and schedules accordingly creating a more robust plan.
Resource Allocation: AI can be used to allocate resources such as vehicles and drivers efficiently. Machine learning algorithms can analyze historical data and predict demand, which can help in deciding the number of resources needed for a particular period.
Customer/Driver Satisfaction: AI can be used to improve customer/driver satisfaction by optimizing the delivery schedules and reducing the delivery time. This can be achieved by analyzing customer/driver behavior and preferences and incorporating them into the VRP algorithm.
Q: What are the limits and risks of AI in VRP context?
Answer An: As businesses turn to artificial intelligence (AI) to optimize vehicle routing problems (VRP), it is important to carefully consider the limits and risks associated with AI implementation. We explore these factors and provide insights to help businesses navigate the potential drawbacks of using AI in VRP.
Limits:
- Data size needed: to learn valuable insights, enormous amounts of historical data is needed.
- Computational Resources: AI requires significant computational resources to perform its calculations, which can become a bottleneck for large VRP instances.
- Data quality: The quality of the data fed into AI models is crucial to their effectiveness. If the data is incomplete, incorrect, or inconsistent, it can lead to inaccurate solutions.
- Complexity of the problem: VRP can be a very complex optimization problem, especially when considering real-world constraints like traffic, weather, and vehicle capacity. The complexity of the problem can limit the effectiveness of AI models in solving it. We see AI approaches yielding satisfying results for simpler cases, these are promising for future evolutions.
- Trade-offs between solution quality and computation time: AI algorithms need to balance solution quality with computation time, especially in real-time applications. Sometimes, the AI algorithm may not be able to find the optimal solution within a reasonable time frame.
Risks:
- Overreliance on AI: An overreliance on AI to solve complex optimization problems like VRP can lead to complacency and reduced innovation in traditional problem-solving methods.
- Black Box Problem: AI models can be difficult to interpret, which makes it challenging to understand why certain routes are recommended. This lack of transparency can make it difficult to identify and correct errors or biases in the algorithm.
- Overreliance on Historical Data: AI models are typically trained on historical data, which can be problematic when conditions change.
Overall, it is important to carefully consider these risks and limits when implementing AI in route optimization to ensure that the benefits outweigh the potential drawbacks.
Q: How does PTV Logistics Addresses Tasks that Competitors Claim to Solve with AI?
Answer An: With AI constantly changing the landscape of the business world, a few of our competitors declare to have achieved mastery in incorporating AI into their solutions. We examine how our company addresses this challenge and endeavors to attain superior results.
- Take for example the statement that one should learn from the manually applied changes to the planned routes by the dispatcher, considering “not everything is known in master data and never will be”.
- In this particular case, we can utilize AI to automatically improve, enhance, or correct the master data, instead of using AI to manipulate the VRP solution. There are two primary reasons behind this approach.
Firstly, our VRP algorithm is specifically designed to generate the best possible solution based on a dataset that is assumed to be complete and accurate. Therefore, tweaking or manipulating the solution may not necessarily lead to improvement since the algorithm already assumes the data is reliable.
Secondly, the “black box” argument emphasizes the importance of transparency in the decision-making process. By understanding why certain routes are chosen, based on an underlying cost model, planners can make informed decisions. Modifying the solution could result in a more expensive plan, providing valuable insights into the algorithm’s decision-making rationale.
Q: What sets our approach apart, and how does it surpass others?
Answer An: We continuously enhance our VRP-solving algorithm through academic research and our own studies. We aim to provide cutting-edge outcomes that exceed industry standards, which we achieve by subjecting our modifications to rigorous benchmarks and comparing them with our competitors. We are proud to say that our algorithm currently delivers exceptional results, and we will continue to innovate and use the right mix of algorithms. Thus, if our algorithm yields an unexpected result, we are inclined to believe that the issue lies with the input data rather than the output. AI could be utilized as a learning tool to study the adjustments made by humans in the past and use that knowledge to ask better questions about the algorithm in the future. This could include improving the input data by suggesting changes or identifying gaps in the algorithm’s capabilities.